Insight / Blog
Why carriers should help retailers identify and target problematic returners
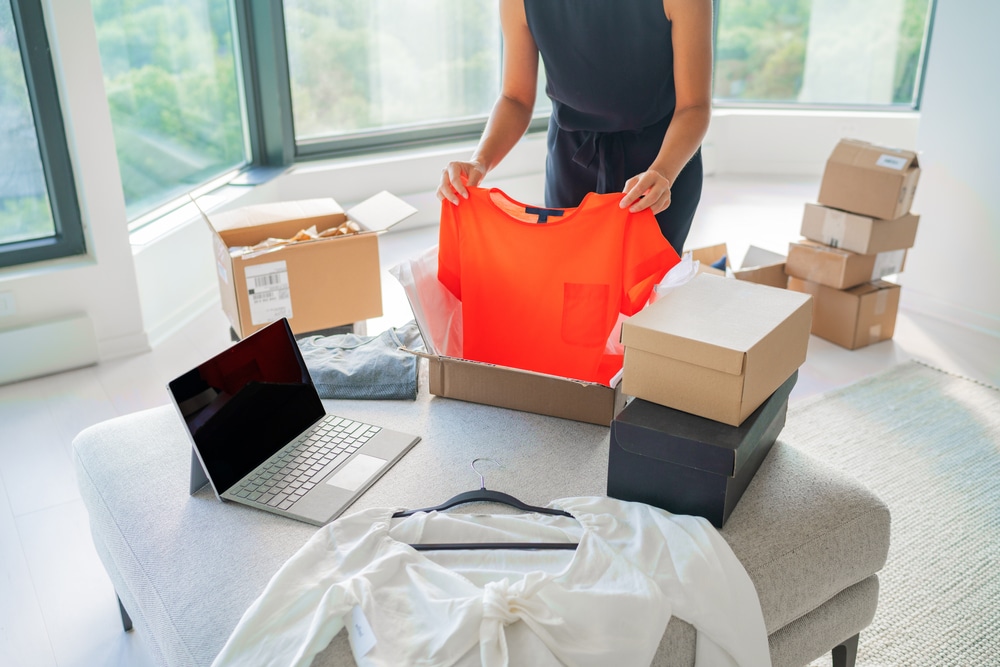
Summary: Become a more valuable retailer partner and secure volume by solving one of ecommerce’s hardest problems.
57% of merchants say that returns are a significant problem for their business, Doddle research shows. One of the biggest headaches for retailers dealing with returns is how to tackle fraud and problematic return behaviours that are costing them the most money.
However, most retailers do not have the data, tech or resources to manage the issue. Our returns report found that 64% of merchants didn’t use an integrated returns solution, meaning that retailers had no control over what was returned, why it was returned, and when, as order data wasn’t checked before the return was made.
If retailers can identify the customers and behaviours most damaging to their bottom line, they can implement strategies to tackle the problem. To do that, they need to segment problem returns and consumers through a returns portals grounded in data, which allows a rules-based approach to decision making – what we call an ‘integrated returns portal’. Most retailers lack the resources to build this in-house, which gives carriers the opportunity to provide a solution. Doing so makes them more valuable ecommerce partners, improving the loyalty of their retailers and helping to attract new business by solving one of ecommerce’s hardest problems.
The Pareto principle – 80% of losses could come from 20% of customers
Returns will always cost retailers, but in many cases, most of the loss attributable to returns comes from a relatively small share of customers. They are likely to be high-frequency shoppers, and so at a first blush they seem like valuable customers – and in some ways they are! However, if they use free shipping offers, buy discounted product and return large proportions of what they buy, the retailer will find they lose a lot of money on this segment very quickly.
Another contributor to margin erosion is fraudulent returns. Similarly to serial returners, only a small minority of the total customer base will engage in this behaviour. The most common type of fraud is wardrobing, where consumers will wear a piece of clothing for a short time before returning the item. Making up 70% of all fraudulent returns, wardrobing costs the retail industry $23.2 billion a year.
As the industry focuses on returns more closely, it’s becoming apparent that the lion’s share of the cost of returns often comes from a disproportionately small share of customers. In ASOS’s latest financial report, the global retailer attributes £100m of loss to just 6% of customers with high return rates, and ASOS’s loss over the reported period was only £60m! The exact figure will vary for different retailers, but if we use the Pareto principle (an assumption that 80% of consequences come from 20% of causes), we don’t think it would be wildly inaccurate to speculate that 80% of returns are generated by 20% of customers.
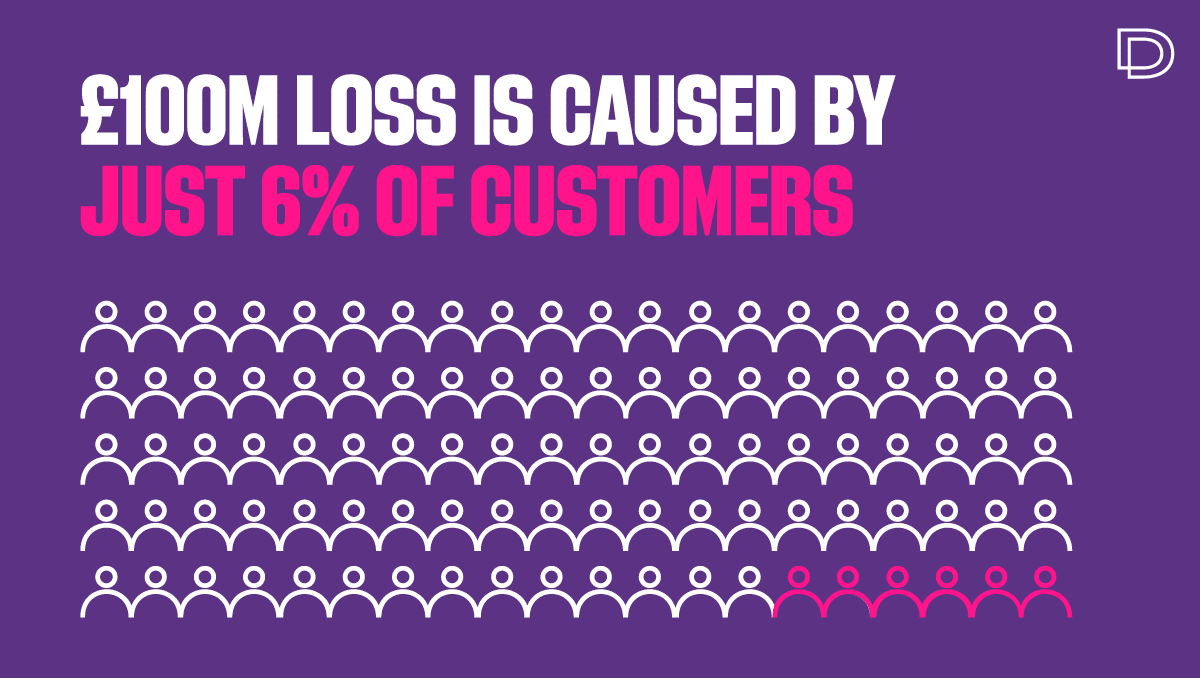
Why ASOS might start charging for returns and what this means for retailers
It’s the retailers’ job to understand which customers might be costing them money.To do that, they need carriers to provide digital returns solutions that provide data on what is being returned, by whom, and why. Most retailers don’t have the resources, tech or time to develop solutions in-house – they need carriers to provide a ready-made answer that they can use to address their biggest issues.
But more importantly, retailers can use returns solutions to understand their data as part of the bigger picture. Not all serial returners are ‘evil’ – some can be fiercely loyal with a long lifetime value. Returns behaviour needs to be properly understood (i.e., what is driving the returns) and put into a longer-term context of customer lifetime value (i.e., the impact of making a return on future purchasing and frequency.) Without this data, no returns algorithm can decide which customers are worth targeting.
By providing access to this data, carrier-provided return solutions are already an invaluable resource to retailers. The extra market advantage comes from allowing retailers to action these insights with specific rules at the point of return.
Helping retailers control returns
Integrated returns portals capture data and reasons for each return, allowing retailers to implement rules and exceptions to tackle the small share of customers who are costing the most in returns. For example, retailers can use the data captured by a digital returns portal to flag serial returners, and implement charges or stricter time rules to ensure returned products are back on the shelves as quickly as possible.
Retailers can also segment returns based on the reason code (why the item is being returned) and the type of item. Amazon is a great example of how these segmentations can be used to determine which returns are paid or free. For example, returns are free if there was a problem with the order where an item wasn’t delivered or arrived broken (i.e., Amazon’s fault). However, if the consumer changes their mind (i.e. the consumer’s fault), consumers are charged to cover the cost. In addition, Amazon’s rules also enable to keep certain items without returning them for a full refund, presumably in cases where the item cannot be resold, or it costs more to be shipped back into stock. With Doddle’s Digital Returns solution, carriers could start providing their retail customers with a similar level of intelligent returns management.
Putting these rules in will help increase profitability, all while providing retailers with strategic information to prevent returns at the source. For example, if the data shows that 70% of returns for one item said that the sizing was too small, retailers could amend the sizing guide on the product listing to tackle returns at the source.
Rather than punish the 20% of customers, retailers could also incentivise them to reduce return rates through discount codes, like the returns initiative from Dress the Population. By offering customers 10% to 70% discounts if they keep the merchandise, the retailer has seen returns fall by 7%, and return costs decrease from $25 to $12 per item returned.
The more carriers help retailers tackle returns, the more they profit.
How carriers benefit from retailer returns
By providing retailers with integrated returns solutions, carriers are helping them to make returns more profitable by dealing with the 20% of consumers making the most loss.
Offering returns solutions strengthens a carrier’s overall value proposition and forms stronger partnerships with existing retail partners. This means capturing a greater share of small and mid-size ecommerce merchants’ returns volumes, potentially adding millions in parcel revenue. That’s because integrated returns solutions won’t completely eradicate returns volume. There will always be returns volume, and by providing retailers with returns solutions, you can exclusively funnel this volume into your channels.
Retailers are looking for solutions for returns. If you’re not the one providing them, they will go elsewhere – and funnel returns volume into the hands of your competitors.
Discover more about why carriers should care about returns with our free report.
Topics:
Related articles
Why mastering returns is key to 3PL growth
With returns management systems, 3PLs can turn returns from a burden to a competitive advantage.
7 tactics to drive self-service parcel drop-off adoption
Focusing on parcel kiosks, we explore 7 tactics to encourage customer adoption and drive volume into out-of-home (OOH) delivery networks.
Lessons from a decade in the first and last mile
A decade as Doddle taught us some lessons - and Blue Yonder helps us see what will matter in the next decade.